Back to all members...
Freddie Kalaitzis
Senior Research Fellow, started 2020
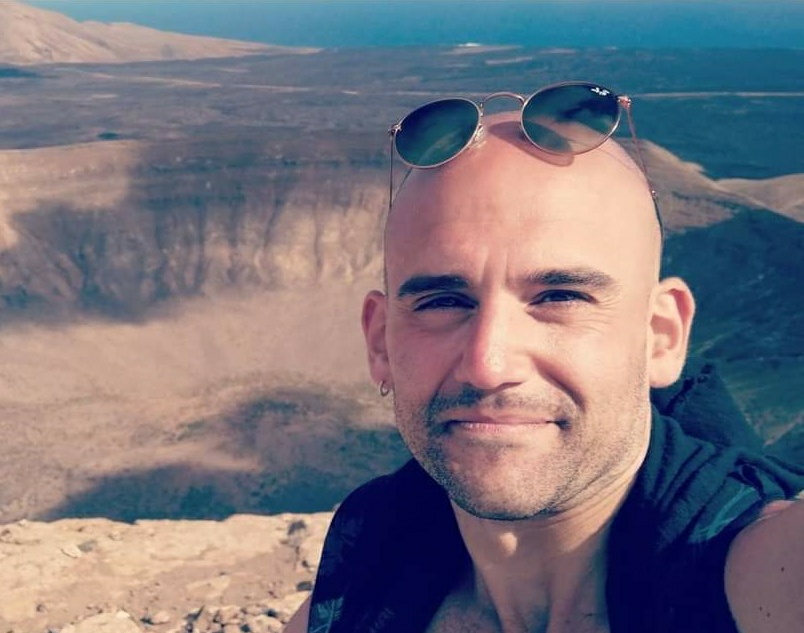
Freddie is a part-time Senior Research Fellow, and Theme Lead of ML for Earth Observation and Remote Sensing, in the Oxford Applied and Theoretical Machine Learning lab (led by Yarin Gal) of Oxford University. He's also an ML & Project Lead at NASA's Frontier Development Lab (FDL), and the (part-time) ML Lead of Trillium Technologies , the R&D production company behind FDL.
Since FDL US 2020, Freddie has been a ML & Project Lead for project Waters Of The United States (WOTUS), in partnership with the USGS, Planet, Maxar, Google Cloud and NVIDIA, towards the ultimate vision for mapping all flowing water on Earth , at near real-time, by fusing LiDAR sensors and daily very high resolution (VHR) satellite imagery.
He started his journey with FDL 2019 as a mentor , helping teams super-resolve solar magnetograms and predict GPS disruptions induced by solar weather .
Until April 2020, he was an Applied Research Scientist in the AI for Good lab (led by Julien Cornebise) of Element AI in London, focusing on applications of ML and statistics that enable NGOs and nonprofits.
During this work, he led the Multi-Frame Super-Resolution research collaboration with Mila Montréal , which was awarded by ESA for topping the PROBA-V Super-Resolution challenge .
He also co-authored the technical report written with Amnesty International, on the first large-scale study of online abuse against women on Twitter , whose front-page coverage in the Financial Times led to Twitter working with Amnesty to better protect the rights of vulnerable users online . Also with Amnesty, he has co-authored a technical blog-post on using VHR satellite imagery to detect evidence of genocide in rural areas of Darfur, Sudan.
Prior to Element AI, he was a Senior Data Scientist in Digital Shadows, a Data Scientist in Microsoft's Xbox EMEA team, and a postdoc in Bayesian statistics (with Ricardo Silva) at the Statistical Science department of University College London . He has a PhD in Computer Science from the University of Sheffield (supervised by Neil Lawrence), and a MSc in Artificial Intelligence from the University of Edinburgh . His PhD research led to contributions in probability methods for dimensionality reduction of data and developed methods for gene-expression time-series to discover genetic factors of disease.
Off work, he occasionally mentors teenagers of ages 12-18 for Teens in AI, and he is the founder of Well-Being in ML , the first official social event at NeurIPS advocating for healthy well-being and mental practice in academia and industry of ML .
News items mentioning Freddie Kalaitzis • Publications while at OATML • Reproducibility and Code • Blog Posts
News items mentioning Freddie Kalaitzis:
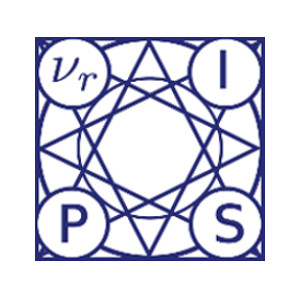
Freddie Kalaitzis to give a spotlight talk at the AI for Earth Sciences workshop at NeurIPS 2020
05 Nov 2020
OATML Senior Research Fellow Freddie Kalaitzis will be giving a Spotlight Talk on Dynamic Hydrology Maps from Satellite-LiDAR Fusion at the AI for Earth Sciences workshop at NeurIPS 2020.
Publications while at OATML:
RainBench: Towards Global Precipitation Forecasting from Satellite Imagery
Extreme precipitation events, such as violent rainfall and hail storms, routinely ravage economies and livelihoods around the developing world. Climate change further aggravates this issue. Data-driven deep learning approaches could widen the access to accurate multi-day forecasts, to mitigate against such events. However, there is currently no benchmark dataset dedicated to the study of global precipitation forecasts. In this paper, we introduce \textbf{RainBench}, a new multi-modal benchmark dataset for data-driven precipitation forecasting. It includes simulated satellite data, a selection of relevant meteorological data from the ERA5 reanalysis product, and IMERG precipitation data. We also release \textbf{PyRain}, a library to process large precipitation datasets efficiently. We present an extensive analysis of our novel dataset and establish baseline results for two benchmark medium-range precipitation forecasting tasks. Finally, we discuss existing data-driven weather foreca... [full abstract]
Christian Schroeder de Witt, Catherine Tong, Valentina Zantedeschi, Daniele De Martini, Freddie Kalaitzis, Matthew Chantry, Duncan Watson-Parris, Piotr Bilinski
AAAI, 2021
[arXiv]
Prediction of GNSS Phase Scintillations: A Machine Learning Approach
A Global Navigation Satellite System (GNSS) uses a constellation of satellites around the earth for accurate navigation, timing, and positioning. Natural phenomena like space weather introduce irregularities in the Earth's ionosphere, disrupting the propagation of the radio signals that GNSS relies upon. Such disruptions affect both the amplitude and the phase of the propagated waves. No physics-based model currently exists to predict the time and location of these disruptions with sufficient accuracy and at relevant scales. In this paper, we focus on predicting the phase fluctuations of GNSS radio waves, known as phase scintillations. We propose a novel architecture and loss function to predict 1 hour in advance the magnitude of phase scintillations within a time window of plus-minus 5 minutes with state-of-the-art performance.
Kara Lamb, Garima Malhotra, Athanasios Vlontzos, Edward Wagstaff, Atılım Güneş Baydin, Anahita Bhiwandiwalla, Yarin Gal, Freddie Kalaitzis, Anthony Reina, Asti Bhatt
Machine Learning and the Physical Sciences Workshop (ML4PS), NeurIPS 2019
[arXiv]
Correlation of Auroral Dynamics and GNSS Scintillation with an Autoencoder
High energy particles originating from solar activity travel along the the Earth's magnetic field and interact with the atmosphere around the higher latitudes. These interactions often manifest as aurora in the form of visible light in the Earth's ionosphere. These interactions also result in irregularities in the electron density, which cause disruptions in the amplitude and phase of the radio signals from the Global Navigation Satellite Systems (GNSS), known as 'scintillation'. In this paper we use a multi-scale residual autoencoder (Res-AE) to show the correlation between specific dynamic structures of the aurora and the magnitude of the GNSS phase scintillations (σϕ). Auroral images are encoded in a lower dimensional feature space using the Res-AE, which in turn are clustered with t-SNE and UMAP. Both methods produce similar clusters, and specific clusters demonstrate greater correlations with observed phase scintillations. Our results suggest that specific dynamic structures o... [full abstract]
Kara Lamb, Garima Malhotra, Athanasios Vlontzos, Edward Wagstaff, Atılım Güneş Baydin, Anahita Bhiwandiwalla, Yarin Gal, Freddie Kalaitzis, Anthony Reina, Asti Bhatt
Machine Learning and the Physical Sciences Workshop (ML4PS), NeurIPS 2019
[arXiv]
Single-Frame Super-Resolution of Solar Magnetograms: Investigating Physics-Based Metrics & Losses
Breakthroughs in our understanding of physical phenomena have traditionally followed improvements in instrumentation. Studies of the magnetic field of the Sun, and its influence on the solar dynamo and space weather events, have benefited from improvements in resolution and measurement frequency of new instruments. However, in order to fully understand the solar cycle, high-quality data across time-scales longer than the typical lifespan of a solar instrument are required. At the moment, discrepancies between measurement surveys prevent the combined use of all available data. In this work, we show that machine learning can help bridge the gap between measurement surveys by learning to super-resolve low-resolution magnetic field images and translate between characteristics of contemporary instruments in orbit. We also introduce the notion of physics-based metrics and losses for super-resolution to preserve underlying physics and constrain the solution space of possible super-resolut... [full abstract]
Anna Jungbluth, Xavier Gitiaux, Shane A.Maloney, Carl Shneider, Paul J. Wright, Freddie Kalaitzis, Michel Deudon, Atılım Güneş Baydin, Yarin Gal, Andrés Muñoz-Jaramillo
Machine Learning and the Physical Sciences Workshop (ML4PS), NeurIPS 2019
[arXiv]
Probabilistic Super-Resolution of Solar Magnetograms: Generating Many Explanations and Measuring Uncertainties
Machine learning techniques have been successfully applied to super-resolution tasks on natural images where visually pleasing results are sufficient. However in many scientific domains this is not adequate and estimations of errors and uncertainties are crucial. To address this issue we propose a Bayesian framework that decomposes uncertainties into epistemic and aleatoric uncertainties. We test the validity of our approach by super-resolving images of the Sun’s magnetic field and by generating maps measuring the range of possible high resolution explanations compatible with a given low resolution magnetogram.
Xavier Gitiaux, Shane Maloney, Anna Jungbluth, Carl Shneider, Atılım Güneş Baydin, Paul J. Wright, Yarin Gal, Michel Deudon, Freddie Kalaitzis, Andres Munoz-Jaramillo
Workshop on Bayesian Deep Learning, NeurIPS 2019
[Paper]
Blog Posts
22 OATML Conference and Workshop papers at NeurIPS 2020
OATML group members and collaborators are proud to be presenting 22 papers at NeurIPS 2020. Group members are also co-organising various events around NeurIPS, including workshops, the NeurIPS Meet-Up on Bayesian Deep Learning and socials. …
Full post...Muhammed Razzak, Panagiotis Tigas, Angelos Filos, Atılım Güneş Baydin, Andrew Jesson, Andreas Kirsch, Clare Lyle, Freddie Kalaitzis, Jan Brauner, Jishnu Mukhoti, Lewis Smith, Lisa Schut, Mizu Nishikawa-Toomey, Oscar Key, Binxin (Robin) Ru, Sebastian Farquhar, Sören Mindermann, Tim G. J. Rudner, Yarin Gal, 04 Dec 2020