DPhil the Future: ChatGPT - a mathematical assistant in the making?
Posted: 8th June 2023
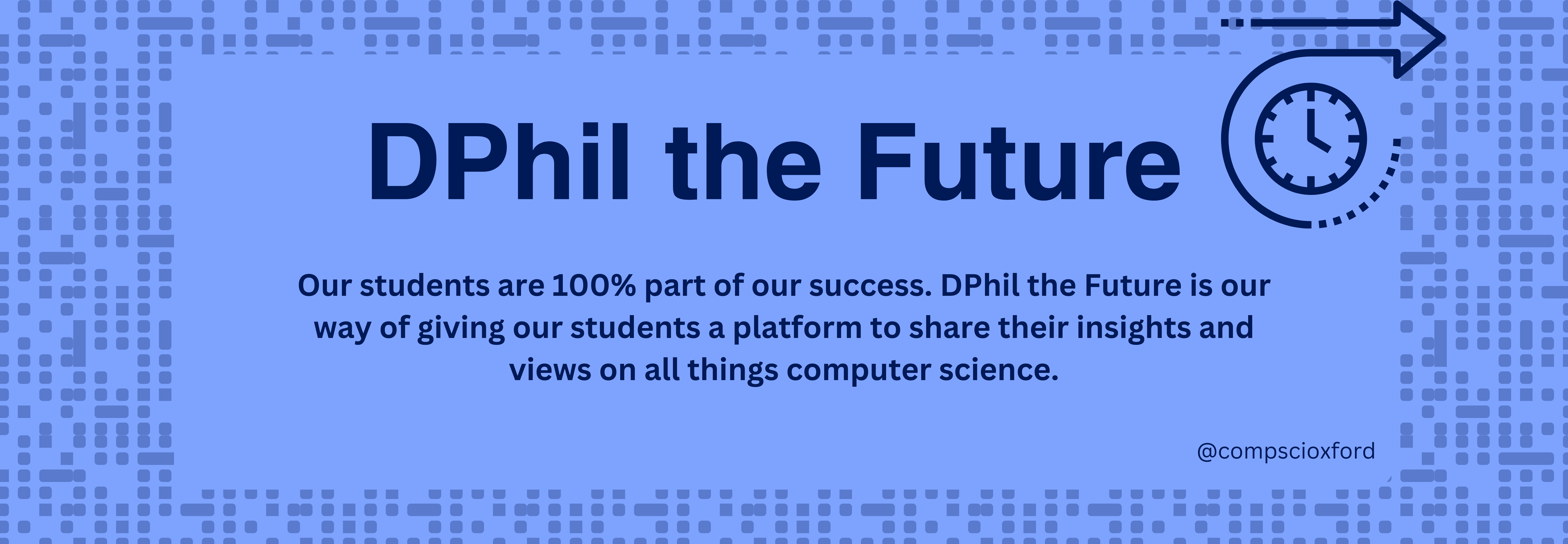
DPhil student Frieder Simon looks at the capabilities and limitations of ChatGPT
The State of Affairs
The remarkable capabilities of ChatGPT and GPT-4 models gave new impetus to the goal of automating mathematics. Traditionally, the automated theorem proving community had attempted to make math amenable to automation by formalising it and searching for proofs. Unfortunately, this decades old approach has not turned out to scale well to graduate-level mathematics and beyond. The rise of language models has led to new efforts to automate mathematics directly using natural language. The arrival of ChatGPT ignited the Twittersphere, which abounds with anecdotal evidence of brilliant answers of ChatGPT – as well as with examples of math gone wrong.
Here is a particularly tricky one: What is the integral of 1/(2 + ln(3/2))? Tricky it is for ChatGPT indeed, as even the latest version (from 3rd May 2023) starts its answer by claiming that ‘the integral of 1/(2 + ln(3/2)) cannot be expressed in terms of elementary functions, so it is an example of a transcendental function’, which - the author hopes - will amuse the readers. For even school children should find this integral to be anything but tricky; recognising that there is no variable in this expression to integrate against means that arriving at the integral only amounts to adding a single variable, say “x” (and an arbitrary constant). ChatGPT’s misstep is likely due to the rarity of such integrals in problem collections, and therefore in its training data.
GPT-4 on the other hand, whose performance on a large batch of standardised exams exceeds that of all previous versions of ChatGPT, manages to solve this integration task correctly! But this ray of optimism after ChatGPT’s atrocious claim is immediately eclipsed by the slightly dubious reasoning GPT-4 provides, which we quote in full: “To find the integral of the function 1/(2 + ln(3/2)), we can first simplify the expression in the denominator. Let k = 2 + ln(3/2). Now the function becomes 1/k, where k is a constant. The integral of 1/k with respect to x is simply (1/k)x + C, where C is the constant of integration. So, the integral of 1/(2 + ln(3/2)) with respect to x is: (1/(2 + ln(3/2)))x + C”. There is still hope for mathematicians to continue their profession. Or is there? Examples such as these call for a rigorous evaluation of the mathematical abilities of ChatGPT.
A New Dataset for Natural Language Mathematics
‘While there are several datasets for mathematical word problems, they lack the comprehensiveness of datasets in more established domains like computer vision or natural language processing.’
Their evaluation schemes rely typically on a simple correct-incorrect classification of the models’ output. This is sufficient for common sense mathematical reasoning - but not enough to test advanced mathematical capabilities, which ChatGPT in some cases seemed to have.
In light of this, the author developed a new type of dataset and rating methodology and organised a team of researchers, comprised predominantly of mathematicians, to help and assist in measuring how well language models can do advanced mathematics.
The new dataset, preliminarily called “GHOSTS”, tests mathematical capabilities on more axes than any of the previous natural-language datasets: completing proofs, searching for theorems, and numerical reasoning abilities are just some aspects that are being tested. This is accompanied by a new methodology, that is more fine-grained than those accompanying previous datasets. To obtain a more nuanced understanding of the models’ capabilities, we rated over 1,500 responses on a scale of 1-5 and assigned various error codes and warning codes to flag certain undesirable reasoning behaviours of the model. These range from flagging whether the model got a bad rating because computations were wrong, proof steps were missing, or edge cases were ignored - to the models providing much more information than was asked for or withholding names of well-known theorems. In a sense, the role between a compiler and a human has been reversed: A human is rating the output of a piece of software and providing error messages and warnings, for input that the software provides.
The 1,500+ responses are split among different Mathematics Subject Classification (MSC) codes, levels of mathematical difficulty (ranging from elementary integration, as illustrated above, to questions similar to mathematical Olympiads), levels of mathematical sophistication (we have included some graduate-level textbooks), whether the data was likely included in the data used to train the model or not - and many more categories.
This is just the first step. We are hard at work to add new datasets, that test further aspects of mathematical reasoning, to improve the methodology, and to evaluate further models in a more automated way. Because this undertaking is too much for a small set of researchers, we are working on letting the community submit datasets and opening up our dataset to let everyone contribute.
Results
‘ChatGPT works best as a mathematical search engine but falls short in proving theorems or performing numerical computations – though occasionally it does surprise!’
For the task of performing numerical computations, the Toolformer approach offers a solution. An instance of this is the collaboration between Wolfram Alpha and OpenAI to allow ChatGPT/GPT-4 to call directly Wolfram Alpha APIs, generate code, and integrate that answer in its output.
Searching for mathematical theorems is a non-trivial undertaking and the model manages to often correctly infer what is being sought (the author learned the name of ‘vague topology’ from ChatGPT). While on numerical or math word problem tasks ChatGPT didn’t perform quite as well as other, older models that were specifically trained to solve these tasks, its performance was not abysmal either.
Since updates to ChatGPT are released almost every month, one interesting thing to consider is how much better each model iteration gets. When comparing the same prompts on the 9 January 2023 version of ChatGPT to the 30 January version, which according to OpenAI’s release notes had “improved factuality and mathematical capabilities”, we see that reality is quite different: The scores do not change in a consistent way between the versions, and largely remain similar.
While a more detailed discussion and figures is available , the overall conclusion is that there is promise in the language-model approach toward automating mathematics, as it is useable already today for selected use cases. There is research underway to unify the formal approach, championed by the automated theorem-proving community with the language model approach.
‘From an educational perspective university teachers can rest assured that (for now) ChatGPT will not render them redundant or invalidate (sufficiently difficult) take-home assignments. Students are also not yet off the hook; if their goal is to use ChatGPT or GPT-4 to pass a university exam, it might just be safer to copy from their average peer!’